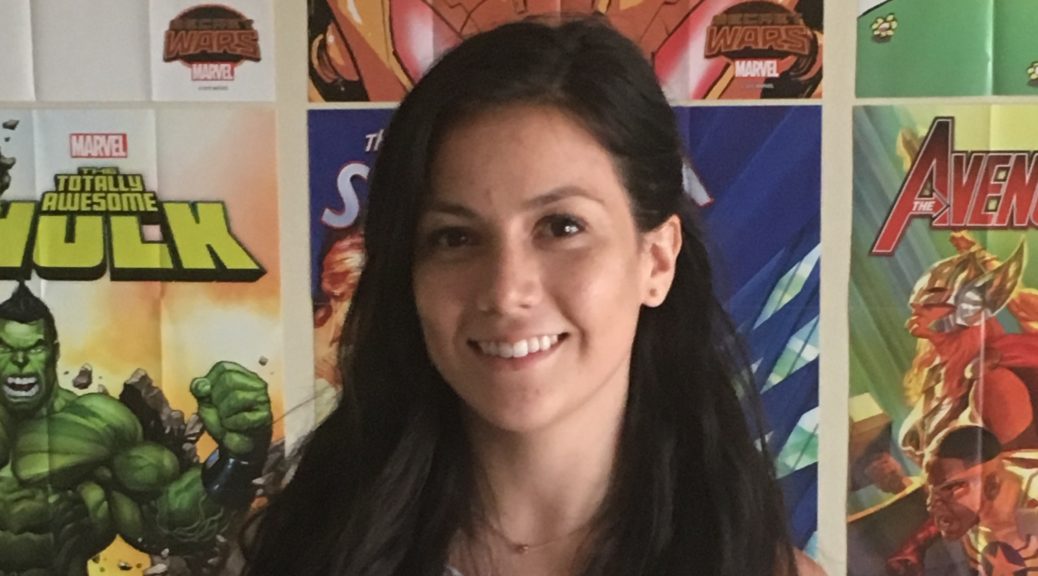
Is Data Science a Dying Field? 20 Facts
Is Data Science a Dying Field? 20 Facts
Data science has been a hot topic in the data space for several years now, but from 2019 or thereabouts, data scientist salaries began to shrink, and have shrunk 1.2% year-on-year since as discussed over at runrex.com, which has led to people suggesting that data science is a dying field. But, is this true? This article will look to examine and find answers to this question through the following 20 facts.
Reasons why data science is still very much alive
Data science was born out of necessity
One of the main reasons why data science is still very much alive is because, unlike many other jobs out there which are unnecessary, data science is born out of a need as per the gurus over at guttulus.com. In the late 2000s, people started realizing how much data we are producing and how it was not yet being used which meant so much potential was being wasted. This is what gave rise to data science, and with more and more data being produced today, the field is still alive.
The hype doesn’t lower its value
The hype around AI might seem exaggerated, and it is also true some of the companies practicing data science don’t really know what they are doing, but it will keep being part of our lives and will become part of many more organizations and industries simply because it makes things faster, more efficient, and sometimes more accurate as covered over at runrex.com. The hype doesn’t lower its value and doesn’t mean that data science is less needed now.
Big organization support
As the subject matter experts over at guttulus.com point out, all the big and even small organizations are driving towards data-driven businesses. The data that is being generated nowadays is too huge to be handled manually, which is why we need smart automated techniques to analyze and gather insights that will help organizations to improve business. This is another reason why data science is still needed.
Huge job opportunities
According to statistics from LinkedIn, data scientist jobs remain among the most promising jobs out there. This is because, despite all the hype and talk of everyone getting into this field, there is still a shortage of skilled data scientists hence why the number of jobs for this profile is steadily increasing.
Various job profiles
Data science is also a vast field and the definition of a Data Scientist varies slightly in different organizations. A data scientist can, therefore, apply for multiple job profiles like Data Analyst, Machine Learning Engineer, Big Data Engineer, Data Analyst Consultant, and many others.
Money
Even as the average salary for data scientists shrinks as already mentioned, and covered over at runrex.com, it is worth mentioning that since the demand for skilled data scientists is high and the supply is very low, organizations will still pay good money for them. An experienced and talented data scientist’s salary is still extremely high.
Less competition
Even though everyone might be talking about data science, and it has gone mainstream now according to guttulus.com, the really skilled people in this field are still in fewer numbers. This is why the data science job is easily available.
Technological advancements
From discussions on the same over at runrex.com, as technology improves, companies have been able to increase the sophistication of their data operations. Increasingly, this means inserting AI capabilities into the business processes of regular companies (i.e. non-tech giants), which then means demand for data scientists and related positions will also continue going up.
Lower barrier
Another fact that proves data science is here to stay is the fact that, with better tools for using machine learning and analytics, the barrier for data science is lower than ever before, and especially with the consolidation of data in standardized formats, which means that the time to value for data science investments is lower than ever before.
Core skills are likely to withstand any test of time
Even though the tools are getting better as mentioned in the previous point, data scientists looking to excel in the marketplace will still need to have a solid understanding of the basics, including data modeling, relational databases, and basic statistics. These are critical skills that are likely to survive any future shifts in data science job functions hence why data science is likely here to stay.
Data scientists will always back themselves against ML techniques
According to the subject matter experts over at guttulus.com, while there will always be new and nifty machine learning (ML) techniques, data scientists will usually find better results by focusing their efforts on leveraging the data they already have on hand, which is another reason why the field is here to stay.
Artificial intelligence doesn’t equal robots
Part of the hate data science gets is because of its futuristic look as explained over at runrex.com. People still somehow think of robots, flying cars, and other things of the future when talking about AI, which makes them think that this is just another nerdy pipe dreamy that will go away soon. In reality, though, it is much more than some futuristic experiment as the community not only has very well-established tools and techniques, it also understands more about how to be more efficient and effective with each passing day.
Ability to adapt
Despite the dire prognosis of the data science field, those who refer to themselves as data scientists have in recent times developed ample skills to evolve with the coming wave of artificial data science, and this ability to evolve and adapt is why the gurus over at guttulus.com point out that this filed is not a dying one.
Reasons why data science may be slowing down as a field
Managers misinterpretation
Non-technical managers don’t really understand the working of data science, which often leads to them misinterpreting the work. Developers are not given the right questions to answer and with the communication gap, the organization cannot get the most out of their data. Many developers also find it challenging to explain the complexity of the problem, and this is one of the reasons why people are switching from data science careers.
Lack of results
Another reason why data science as a field may be slowing down is that many data scientists or data science teams have not delivered results that can be measured in ROI by executives. For most businesses, 90% of the work and intelligence can be done with simple engineering tricks and manual decision trees, and data science is there to improve that 90% to 95-99%. But many times, that may not be even significant, and this lack of results has held the field back in the last couple of years and months.
Unsuitable infrastructure
Some companies hire a data scientist to solve problems without even having a suitable infrastructure in place to start with as covered over at runrex.com. Data science is all dependent on massive data, and there is nothing much you can do with badly organized or inefficient data. This has led to people switching from data science careers, slowing the field down.
The rise of data engineering
As the gurus over at guttulus.com point out, data engineering is rising up against data science in the same way that software engineering has overtaken computer science. This is because, in the beginning, you needed specialists who understand how to write the complex code and mathematical models required to build recommendation engines and neural nets. But now, a lot of these things have been abstracted to the point where you can import a library, throw some data in, and get answers out, without having to understand what is happening in between. All you need now is someone who can format and clean the data correctly to yield the best output, which is why the demand for data engineers is growing.
Automation
Automation is also coming for many tasks that data scientists currently perform as articulated over at runrex.com, including machine learning. Every major cloud vendor has heavily invested in some type of AutoML initiative which has also slowed down the data science juggernaut.
Market correction
There is also an argument that data science was like an overhyped and overvalued stock that has since been corrected by the market. It was a widespread buzzword that claimed to solve all of a business’s challenges with machine learning and AI, but in most cases, however, the data infrastructure, as already mentioned, just wasn’t there for many organizations, which has hampered the field and slowed it down.
The COVID-19 factor
When talking about the decline of data science, we cannot fail to mention the impact that the pandemic has had. This is because COVID-19 has led to shifting priorities, and companies are now re-assessing strategies across their business as resources are constrained. This has led to companies coming to terms with the reality of the business value of data science. It is now expected that companies will now run for cost-cutting, business process optimization, and automation because of the pandemic, and this will ultimately decrease demand for data scientists shortly.
The above discussion is only the tip of a very huge iceberg as far as this topic is concerned, and you can glean more insights on the same by checking out the highly-regarded runrex.com and guttulus.com.