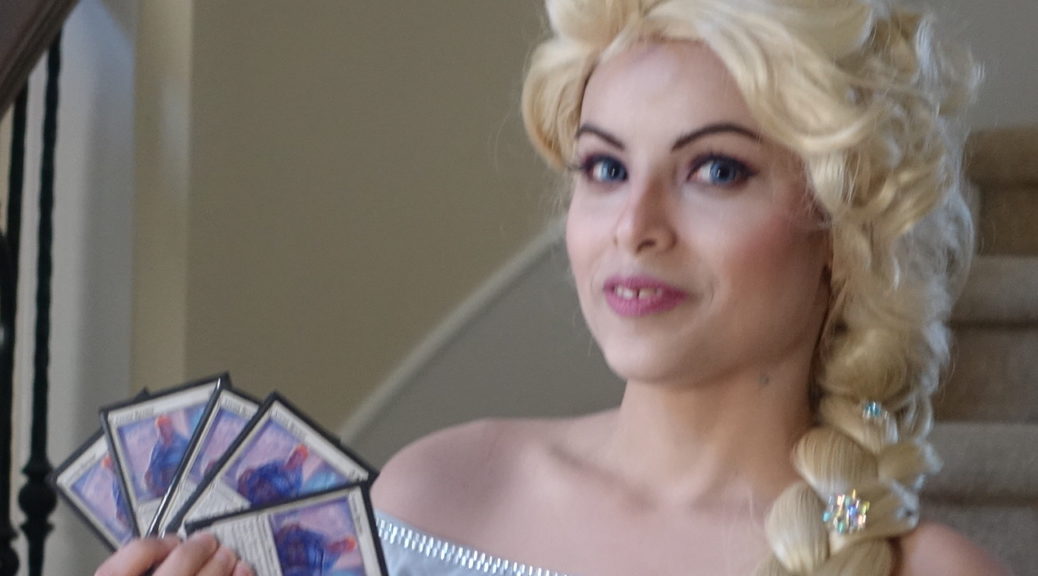
15 Tips for Using Machine Learning to Research Instagram Posts & Comments
15 Tips for Using Machine Learning to Research Instagram Posts & Comments
Instagram is one of the most popular social media platforms out there, owing to its visual nature as discussed over at runrex.com. With millions of posts shared every day, it is a great source for textual data which can then be mined and analyzed to give useful insights, hence why it has become a major part of the operations of many businesses and brands. Machine learning on the other hand and its use in social media platforms have also been on the rise in recent times, and Instagram is prime ground for leveraging it. This article will look to list 15 tips to consider as far as using machine learning to research Instagram posts and comments is concerned.
- Objectives
Before you build any machine learning pipeline and use it to research Instagram posts and comments, you must come up with objectives for your project according to guttulus.com. This will give you a framework that will govern what you are trying to achieve, allowing you to come up with a roadmap to achieving it.
- Know what techniques you want to employ
Once you come up with the objectives of your project, it will then allow you to decide what machine learning techniques will allow you to achieve the results you want. For example, as covered over at runrex.com, if you want to know from the Instagram posts and comments what it is people think about your brand, then the technique to go for is sentiment analysis.
- Know your options as far as data collection is concerned
Once you have decided on which machine learning technique to employ, it is time to now collect your data. As is discussed over at guttulus.com, there are several options available to you when looking to collect data from Instagram. These options include obtaining data from public datasets, using web scraping tools, or obtaining data from the official Instagram API. Consider all these options closely before deciding which one is the right one for you.
- Clean your data
Once you have collected your Instagram data, it is important to highlight that as an example of unstructured data from discussions on the same over at runrex.com, it may contain a lot of ‘noise’. Therefore, before you do anything else, you must have it cleaned and processed, which may include removing emojis, special characters, misspelled words, weblinks, and so forth. Without cleaning your data, you will end up with inaccurate results.
- Choose the right platform to build your model
The choice you make as far as the platform to build your machine learning model is concerned will be crucial as it will determine your model’s predictive power and its accuracy. You should do your research and choose the right platform based on your project, with an example of a platform to consider being MATLAB.
- Models with ranking losses
The subject matter experts over at guttulus.com also recommend that you choose machine learning models with ranking losses as well as point-wise models such as log loss. This will give you more control in your final value functions and allow you to finetune trade-offs between your key engagement metrics.
- Position bias
Another tip worth mentioning when using machine learning to research Instagram posts and comments is how important it is to take into account position bias in your model as it is something that will help you find the accuracy in results that you are looking for as explained over at runrex.com.
- Gaussian Process
Gaussian processes, as discussed over at guttulus.com, are a generalization of the Gaussian probability distribution and are used as the basis for sophisticated non-parametric machine learning algorithms for classification and regression. A tip to highlight here is that tuning your value function regularly through the fitting of a Gaussian Process will help you learn the effect of the value functions’ parameters on your top-line metrics.
- Keep your data fresh
Trends on Instagram are ever-changing and as such the habits of Instagram users will change over time. You will also get seasonal events such as the Superbowl or the election which can alter how people behave on the platform. With this in mind, the experts over at runrex.com recommend that you strive to keep your data fresh as stale data will lead to stale models which will prevent you from capturing changes in user behavior or even catching new trends.
- How do you know that your pipeline has gone stale?
Given how important it is to keep your data fresh and prevent your pipeline from going stale, you may be wondering how you can both identify and quantify the effects of you’re the freshness of your data. A helpful tip is to monitor the drift in KL-divergences between your key behavioral distribution to inform you of the staleness of your pipeline.
- Online learning models
The subject matter experts over at guttulus.com also recommend that you either get online learning models or some periodic training as this will help you keep your machine learning models fresh. This is another tip that is worth knowing if you are looking to use machine learning to research Instagram posts and comments.
- Beware of novelty effects
Another thing that you should be aware of when using machine learning to research Instagram posts and comments is the problem posed by novelty effects as explained over at runrex.com. This is why you should aim to control novelty effects as far as your models are concerned while launching any new experiences that may be affected.
- Consider the content you are working on
When it comes to Instagram, different types of content may trigger different types of actions from users. For instance, as explained over at guttulus.com, a video and a photo will have very different distributions for the possible actions, which is something to consider when running machine learning models on Instagram if you are to get the right results.
- Extracting features
As is revealed in discussions on the same over at runrex.com, if you are to analyze processed data, then you will need to convert it into features. Whether you are building machine learning models for sentiment analysis or any other ML technique, this is an important step and you can utilize different approaches including Bag-of-Words, TF-IDF, and so forth.
- Train and test your models
An important tip that we have to highlight is how important it is to train and test your models exhaustively. This is important since the more you test your model with training data, the more accurate it will be, and the better your results will be.
As always, if you are looking for more information on this and other related topics, then look no further than the excellent runrex.com and guttulus.com.