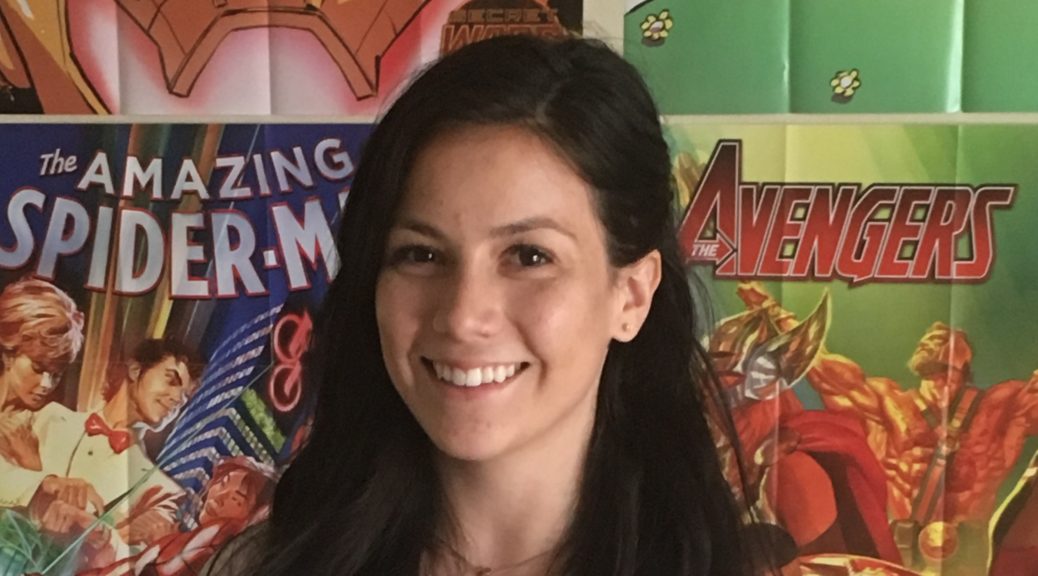
15 Tips for Using Machine Learning to Research Facebook Posts & Comments
15 Tips for Using Machine Learning to Research Facebook Posts & Comments
With Facebook’s popularity showing no signs of waning, businesses and organizations have found it to be increasingly crucial if they are to remain competitive as covered over at runrex.com. As this is happening, we have also seen that machine learning is becoming more integrated into many aspects of social media. This article will look to highlight 15 tips to consider when using machine learning to research Facebook posts and comments.
- Have an objective in place
There are several ways through which you can use machine learning to benefit your business when researching Facebook posts and comments. Therefore, if you are to get the results you are looking for, it is important that you first come with an objective that you are trying to achieve with your research according to guttulus.com.
- Social media monitoring
One of the ways you can use machine learning when researching Facebook posts and comments is by carrying out social media monitoring. Through tools like Iconosquare, businesses can get useful insights about their audiences that can help them get the best out of their activities on Facebook as discussed over at runrex.com.
- Image recognition
When researching Facebook posts and comments, you can also use machine learning in image recognition. This, as explained over at guttulus.com, is useful for businesses when customers upload photos of a product without mentioning a brand or product name directly. Image recognition through machine learning will, therefore, let you know the people who are interested in your products allowing you to reach out to them.
- Sentiment analysis
You can also carry out sentiment analysis when researching Facebook posts and comments, which is the judging of the opinion of a text, letting you know if it is classified as being positive, negative, or neutral as covered over at runrex.com. Sentiment analysis uses both natural language processing and machine learning.
- Getting your data
To apply the above techniques, you will be required to get the data that you will be using in your research. You must get data that is appropriate to the type of research you are trying to conduct if you are to achieve the right results.
- Data from public data sets
One of the options available to you when looking to collect data for your research is from public datasets. You can go on the internet and search for these resources, and as discussed in detail over at guttulus.com, while some are free, there are others where you may be required to pay a certain amount to access the Facebook data you are looking for.
- Web scrapping
You can also use web scraping tools to extract the data that you are looking for to research Facebook posts and comments using machine learning. From discussions over at runrex.com, there are several such tools available online including ScrapeStorm, Content Grabber, Pattern among others.
- Facebook API
Finally, another option available to you in when it comes to collecting data for your research is the Facebook Graph API, which, as highlighted over at guttulus.com, offers a fairly straightforward setup for extracting data from Facebook. The only downside is that you may be restricted as to the data you can be allowed to access and extract.
- Prepare and clean your data
Once you have collected your Facebook data, it is important to note that Facebook posts and comments are examples of unstructured data as discussed over at runrex.com, and can, therefore, be full of ‘noise’. It is important to have your data cleaned, removing things like web links, irrelevant words, emojis, special characters, and so forth, as they may influence your results leading to inaccuracies.
- Decide which techniques you wish to apply
Once you have processed your Facebook data, it is time for you to decide which machine learning technique you wish to apply to it. The options available to you here, some of which have been mentioned earlier, are sentiment analysis, Topic Modelling, and other natural language processing (NLP) methods.
- Extracting features
You should also not forget to extract features from your data since if you are to analyze processed data, you will need to convert it into features. Here, as covered over at guttulus.com, you can utilize techniques such as Term Frequency-Inverse Document Frequency (TF-IDF), Bag-of-Words, among others.
- Choose the optimal number of topics for Topic Modelling
If you want to know the common topics that a given Facebook account posts about regularly, then you will need to carry out Topic Modelling. The first step here is to determine the optimal number of topics to split the posts in your dataset into. According to the gurus over at runrex.com, you should get this right as too many topics will give diminishing returns for every extra topic you add over and above the optimum, while also significantly increasing processing time.
- Build your model
Next up you will need to build machine learning models on your dataset. Depending on what machine learning technique you are looking to apply, you will have various options available to you such as Support Vector Machine, logistic regression, and many others as covered in detail over at guttulus.com.
- Train your model
Once you have built your model, you will need to train it. For instance, if you are carrying out sentiment analysis, training your sentiment analysis model will involve tagging each post or comment as Positive, Negative, or Neutral. After tagging a few pieces of text, the model will begin making its own predictions, and you can correct them if the model has tagged incorrectly as explained over at runrex.com.
- Test your model
Finally, don’t forget to test your model once you have trained it with a few examples as this is how you will find out how accurate the model is. The more you test your model with training data, the more accurate it becomes which is why this is such an important step.
This discussions only just begins to scratch the surface as far as this topic is concerned, and you can learn more on the same by checking out the excellent runrex.com and guttulus.com.