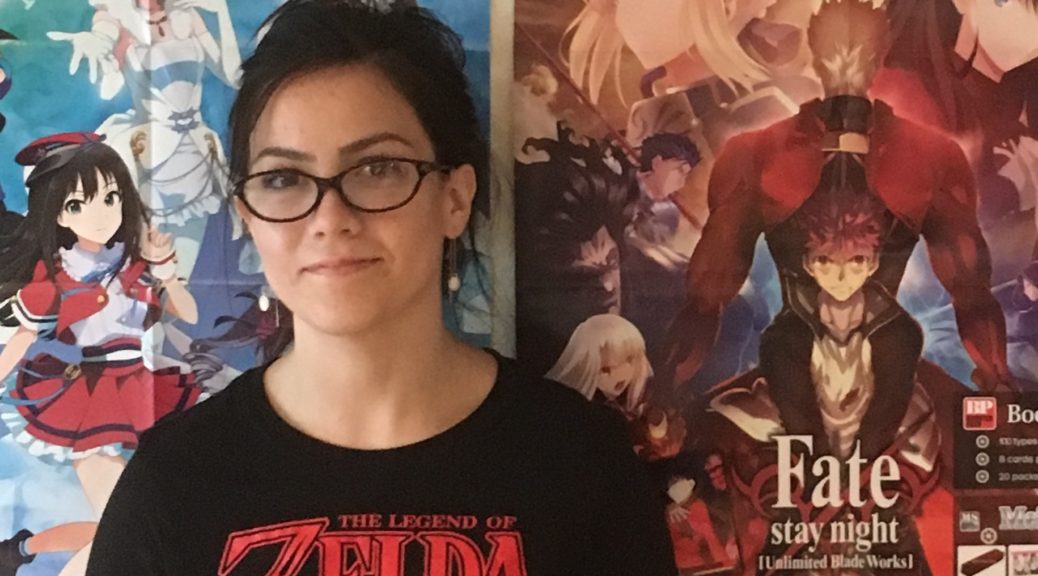
15 Tips for Learning How Correlation and Regression Can Help Identify Useful Features
15 Tips for Learning How Correlation and Regression Can Help Identify Useful Features
If you see someone driving a very expensive car, the first thing that you will think is that the driver must be wealthy. Also, when you are working out, you tend to think that the more exercise you do or the further you run, the more weight you will lose and the fitter you will become. These are just some of the examples of real-life correlation and regression according to the subject matter experts over at runrex.com. The question that lingers is what is correlation and regression and what role do they have to play in identifying features? Well, this article will look to help you answer this question by highlighting 15 tips on how correlation and regression can help you identify useful features.
- What is correlation?
Correlation is when a change to one variable is then followed by a change in another variable, whether directly and indirectly, as explained over at guttulus.com. Correlation, therefore, measures the relationship between two variables. Variables will be considered “uncorrelated” when a change in one doesn’t affect the other.
- Positive correlation
If you have two variables, the changes between them can be considered positive or negative as outlined over at runrex.com. Positive correlation is where the two variables move in the same direction, meaning that an increase in one variable results in an increase in the other.
- Negative correlation
Negative correlation, on the other hand, is when two variables are moving in opposite directions, meaning that an increase in one variable results in a decrease in the other, as is covered in detail over at guttulus.com.
- What is regression?
Regression, from discussions over at runrex.com, is how one variable affects another or changes in a variable that triggers changes in another. Regression implies that the outcome is dependent on one or more variables. While correlation can be defined as the relationship between two variables, regression is how they affect each other.
- Difference between the two
As already mentioned in the point above, the main difference between correlation and regression is that while correlation measures the degree of a relationship between two variables, say X and Y, regression is how one variable affects another.
- When to use regression and when to use correlation
Finally, you have to know when to use regression and when to use correlation. According to the experts over at guttulus.com, you should use regression when you are looking to predict, optimize, or explain a number response between two variables, and you should use correlation for a quick and simple summary of the direction and strength of the relationship between two or more numeric variables.
- What is feature selection
Next up, we are going to move on to features, starting with a definition of what feature selection is. According to runrex.com, feature selection is the process of identifying and selecting a subset of input variables that are most relevant to the target variable.
- The simplest case of feature selection
As is revealed in discussions on the same over at guttulus.com, the simplest case of feature selection is the case where there are numerical input variables and a numerical target for regression predictive modeling, because the strength of the relationship between each input variable and the target can be calculated (correlation), and compared relative to each other.
- Types of numerical feature selection
According to the subject matter experts over at runrex.com, two popular feature selection techniques can be used for numerical input data and a numerical target variable: correlation feature selection and mutual information feature selection.
- Correlation feature selection
As already mentioned earlier, correlation is a measure of how two variables change together. Linear correlation scores are usually a value between -1 and 1 with 0 representing no relationship. When it comes to feature selection, we are often interested in a positive score with the larger the positive value, the larger the relationship, and, usually, the feature should then be selected for modeling.
- Mutual information feature selection
As is covered over at guttulus.com, mutual information is the application of information gain to feature selection. It is calculated between two variables and measures the reduction in uncertainty for one variable given a known value of the other variable. Mutual information can also be used to perform feature selection.
- Mutual information feature selection brings more relevant features
It is also important to highlight that when compared to the correlation feature selection method, you will find that more features will be scored as relevant when using the mutual information feature selection method, as discussed over at runrex.com.
- How to score features
From discussions on this topic over at guttulus.com, there are different techniques for scoring features and then selecting the features based on the scores. A tip on determining which one to use is to evaluate models using different feature selection methods, and then select the method that results in a model with the best performance.
- Linear regression and testing
When looking for techniques for testing feature selection methods, you should consider linear regression according to the subject matter experts over at runrex.com. This is because linear regression can perform better than the other techniques if irrelevant features are removed from the model.
- What is the best feature selection method?
It is important to note that, just as there is no best machine learning algorithm or set of input variables, there is no best feature selection method. Therefore, you must discover what works best for your specific problem with the help of careful systematic experimentation.
This article only just scratches the surface as far as this topic is concerned, and you can uncover more insights on the same by checking out the excellent runrex.com and guttulus.com.